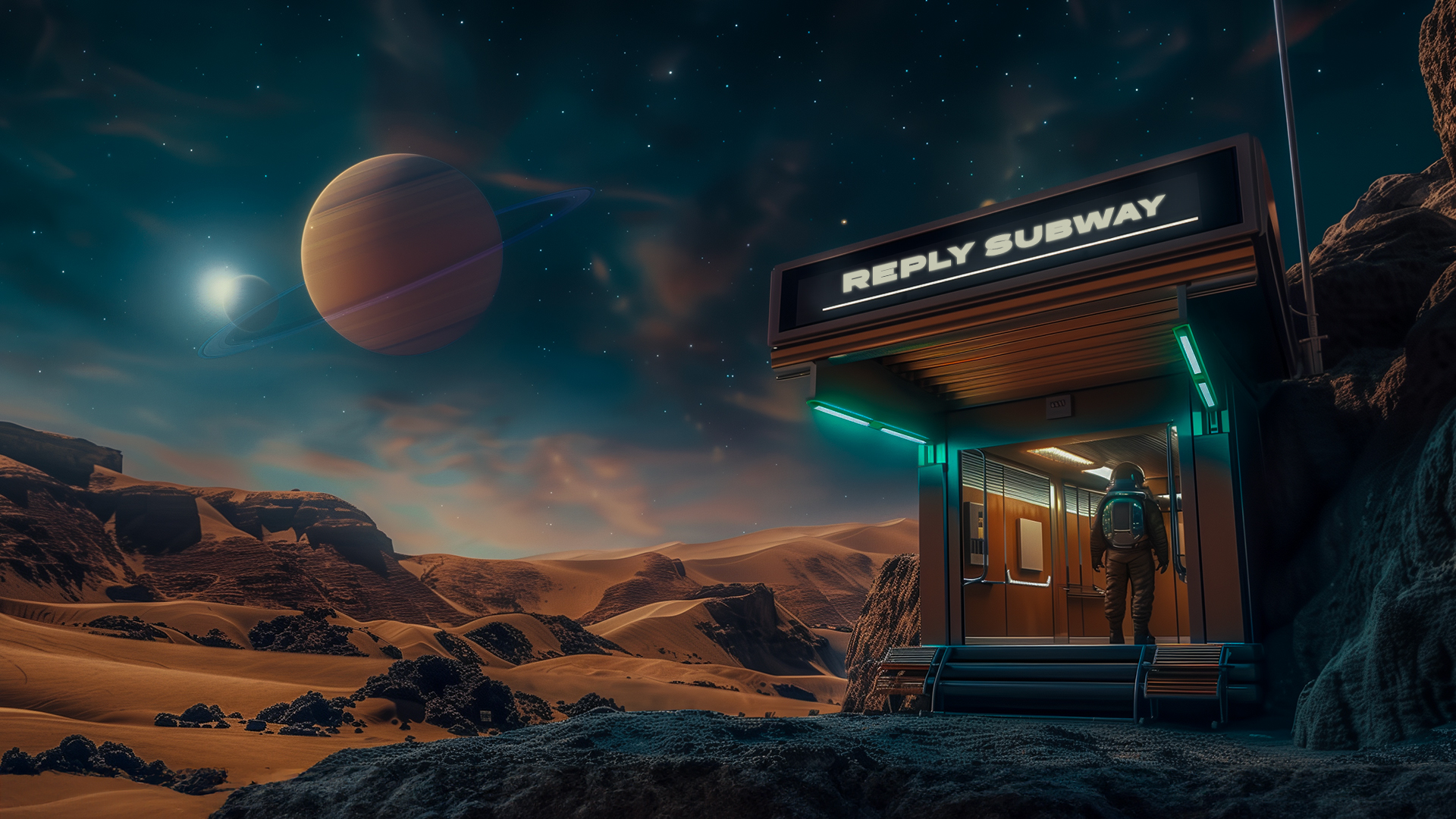)
Artificial Intelligence
Working with AI can improve human-led activities, not just reproduce them. AI can change every business and how we perform almost every task.
)
Prebuilt AI Apps
Generative AI tools
AI as a powerful mind
We dive deep into generative AI and unsupervised AI to create new engines, empowered by their own capabilities and able to imitate not only what humans do, but how they think.
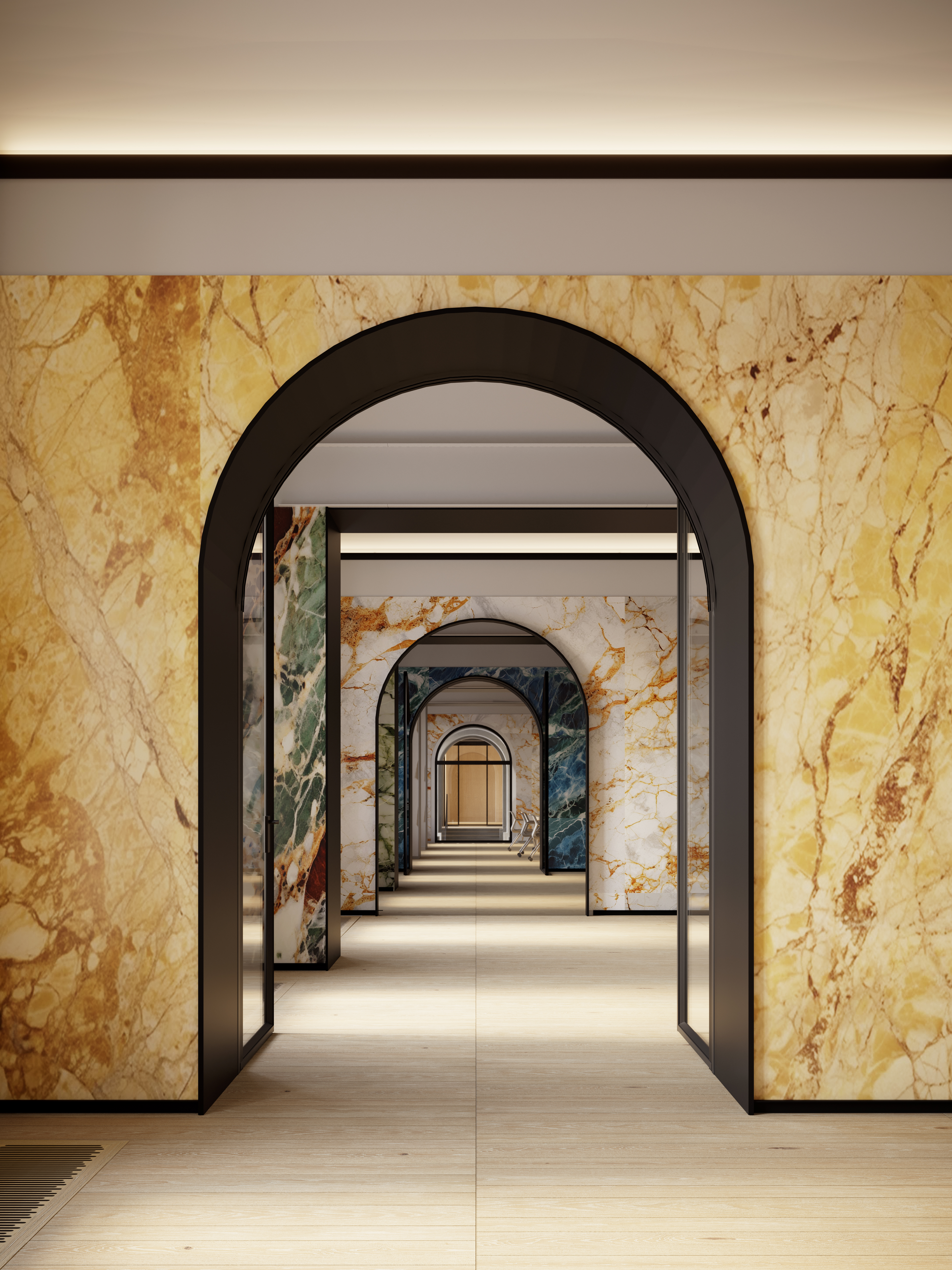;Resize,width=1660)
A new era in design: generative AI and interdisciplinary creative synergy between Reply, ACPV ARCHITECTS, and Marazzi
Premiering in Milan during Design Week, "A new AI generation Marble", where technology, design sensibility and sustainability come together, opening up a future full of new possibilities.
;Resize,width=660)
Reply AI Challenges 2025
Solving technical challenges with AI
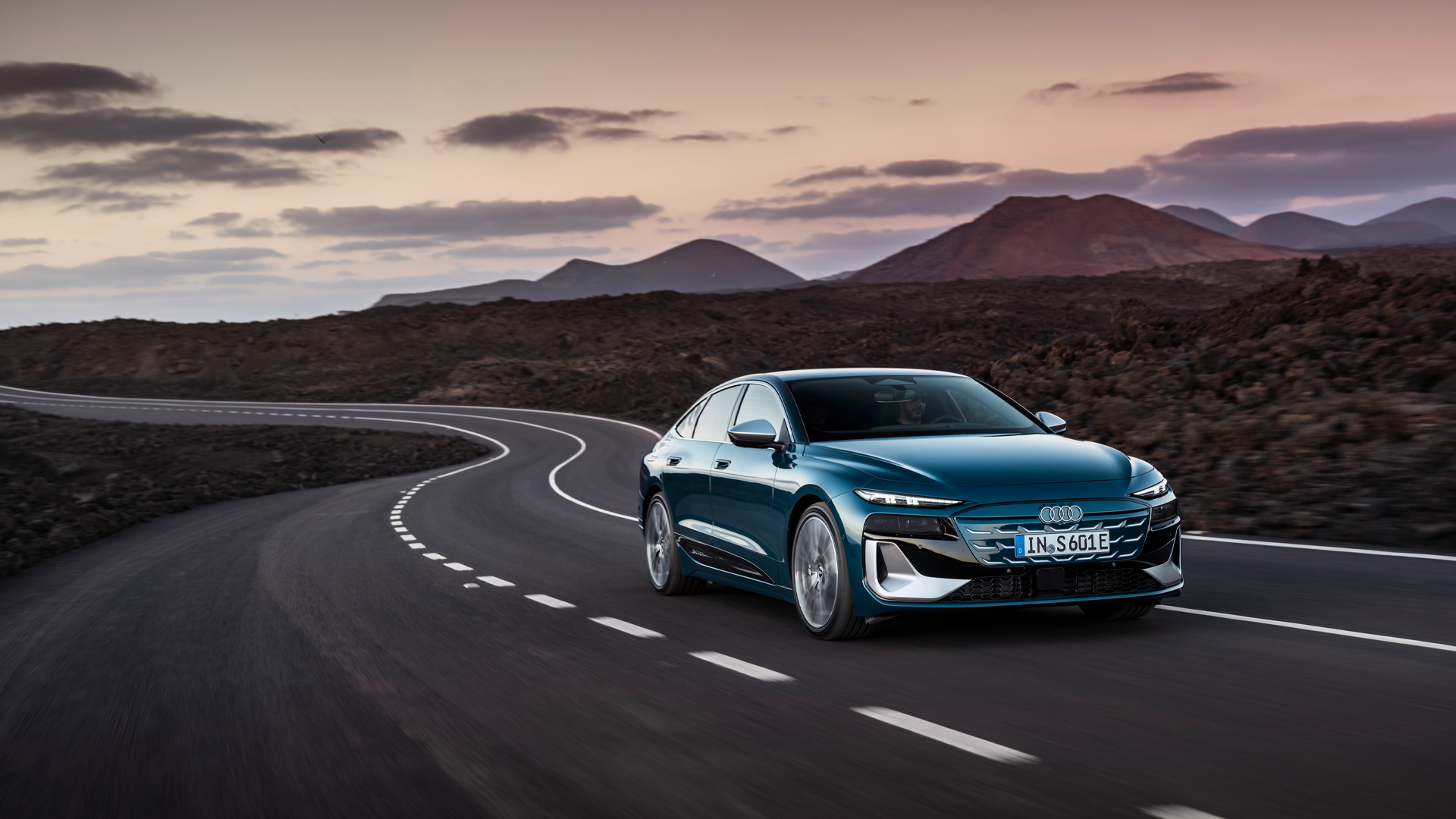,allowExpansion;Resize,width=1660)
)
Devbot: Audi’s intelligent multi-agent system for smarter and safer cloud use
Developed by Audi and Storm Reply, devbot is an advanced Agentic AI solution that intelligently optimizes Audi’s cloud infrastructure – quickly, securely, and reliably.
Improve quality & maintenance with AI
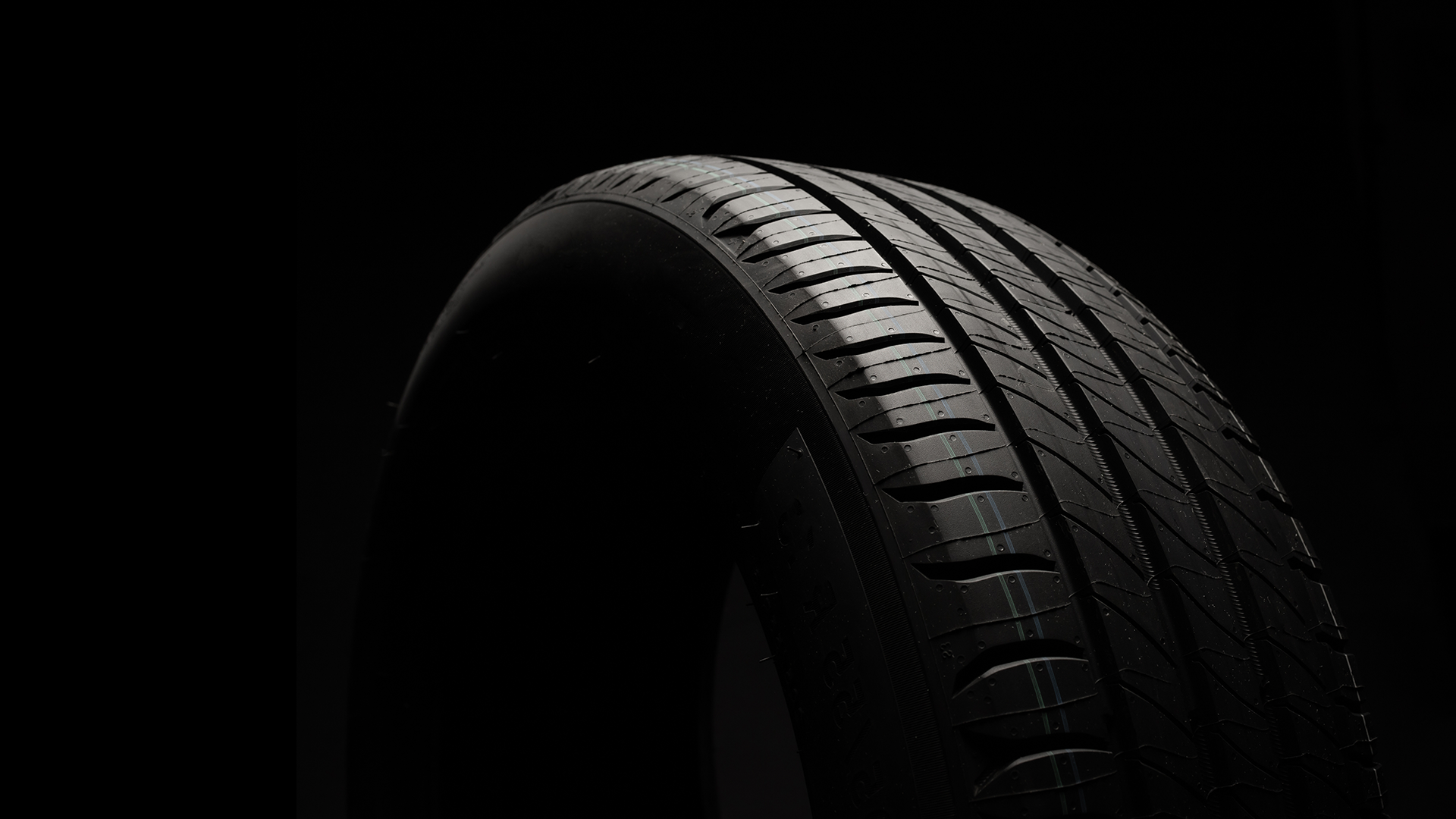,allowExpansion;Resize,width=1660)
)
Predictive monitoring for Michelin's production lines
Sensor Reply has supported Michelin in monitoring complex production lines, developing a scalable and flexible approach, based on AI models, to promptly identify possible causes of failures and prevent production stoppages or inefficiencies.
AI for Business Automation
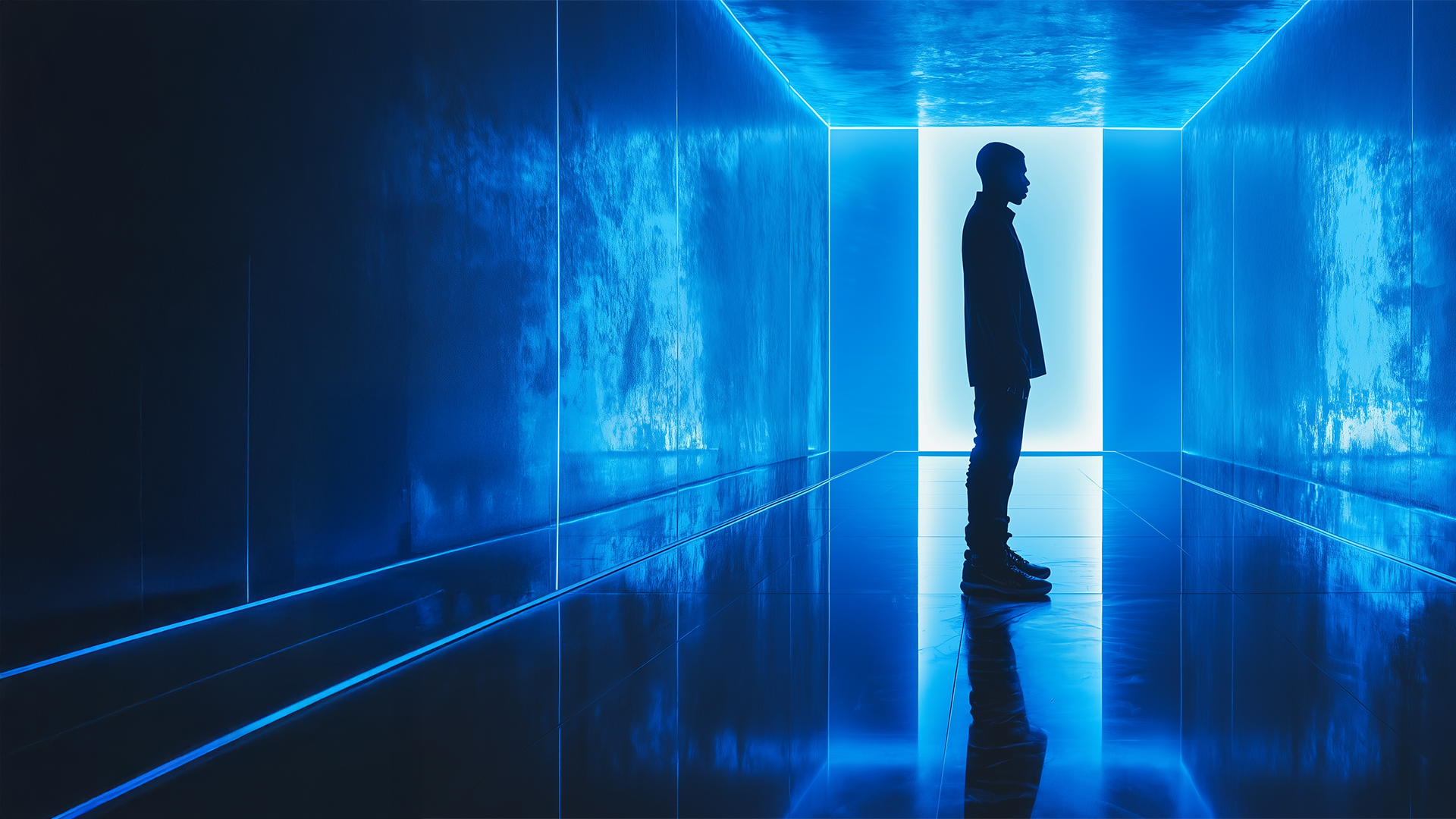;Resize,width=1660)
AI-Powered Procurement
The significant efficiency introduced by Artificial Intelligence in Procurement is opening the door to new efficiencies while enhancing daily support for buyers
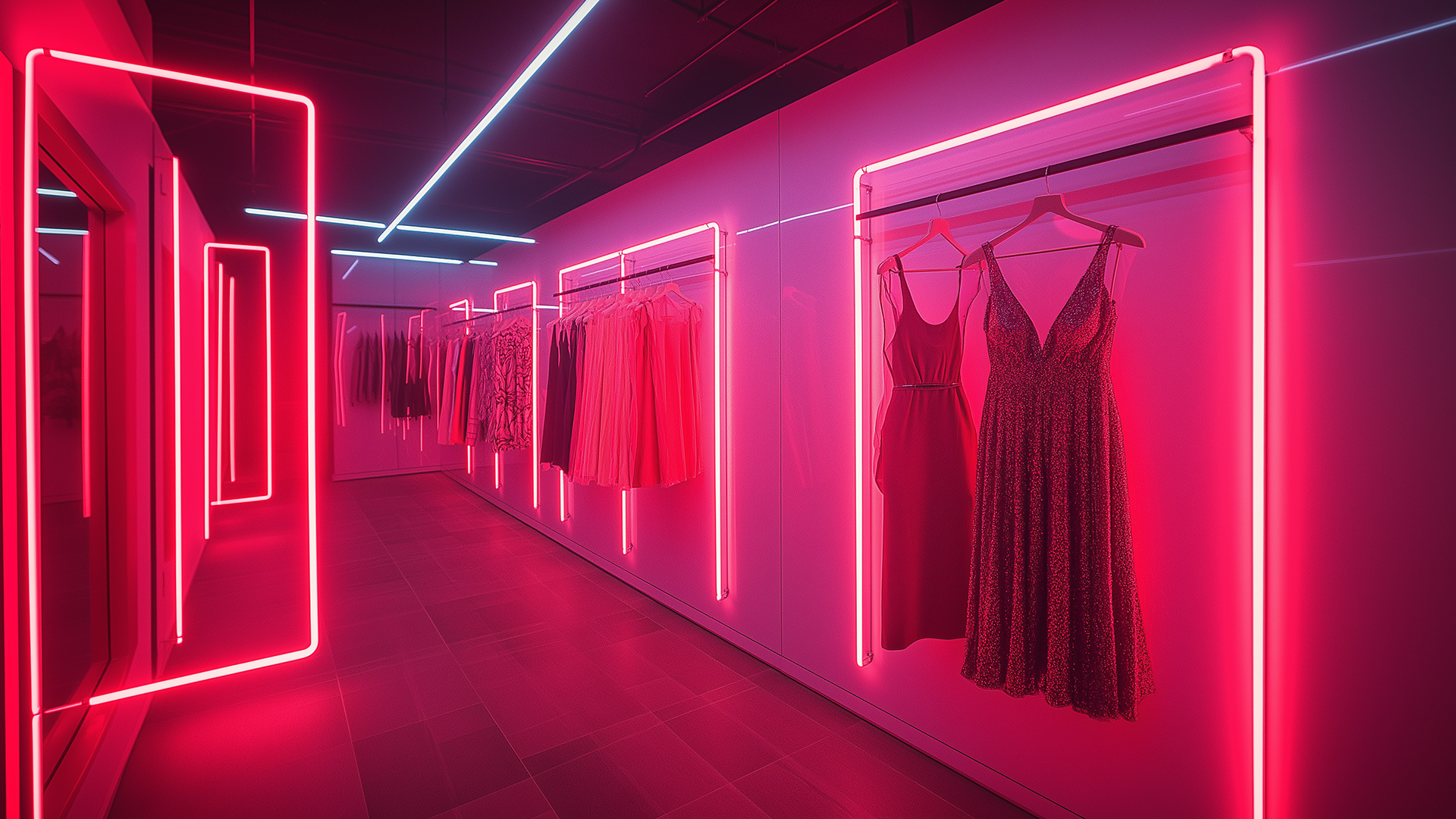;Resize,width=660)
Improving Retail In-Store Efficiency with AI-Powered Agents
Reply’s approach integrates in-store operations under a single layer, to streamline in-store workflows, and enables real-time insights for client advisors and managers.