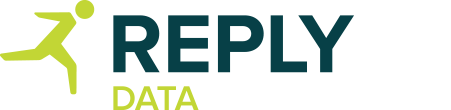
Data Reply is a Reply Group company, a premier AWS partner, offering a broad range of advanced analytics, AI ML and data processing services. We operate across different industries and business functions, enabling our customers to achieve meaningful business outcomes through effective use of data, accelerating innovation and time to value.